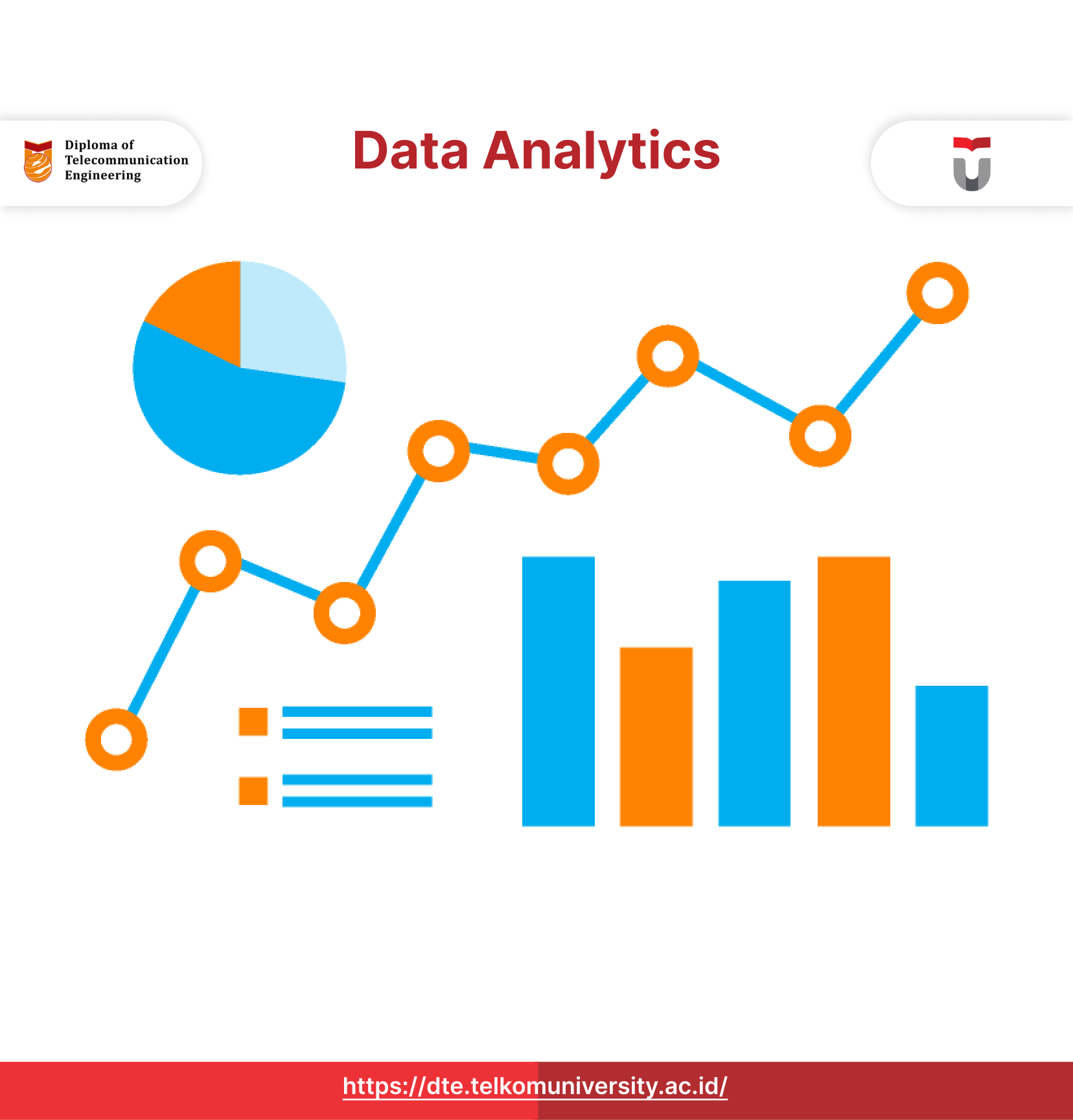
Data Analytics: Definition, Types, Stages, and Benefits
Data analytics is the process of transforming raw data or Big Data into clear and useful information, which can be applied in various sectors, including business, health, education, and scientific research.
In today’s digital era, data has become one of the most valuable assets for companies, organizations, and individuals. Previously hidden information can be analyzed and transformed into invaluable insights thanks to advances in technology and computer science. One of the most important concepts in data processing and interpretation is data analytics . In this article, we will discuss in depth what data analytics is , its various types, its benefits, and how the process is carried out to help companies or individuals in making better decisions.
What is Data Analytics?
Data analytics is the process of transforming raw data or Big Data into information that can be understood and used in various fields, such as business, health, education, and scientific research. In a world that is increasingly dependent on data, analytics is the process of not only collecting information but also finding patterns, correlations, and insights that can be used to make better decisions. In this process, various statistical techniques, computer programming, and algorithms are used to explore, analyze, and present data so that it is easier to understand.
Why is Data Analytics Important?
At the most fundamental level, data analytics enables organizations to:
- Maximize decision making based on valid evidence rather than relying solely on assumptions.
- Improve operational efficiency by identifying ways to reduce waste or improve processes.
- Improving the quality of customer service can be achieved by deeply understanding their needs and preferences.
- Innovation of new products or services based on insights gained from data analysis.
- Reduce risk by predicting potential problems or finding ways to overcome existing challenges.
Additionally, data analytics is an integral part of a larger global trend of leveraging technology to advance innovation and efficiency across sectors. From business to healthcare, data analytics is key to providing sharper insights for decision-making.
Benefits of Data Analytics, Why is it Important for Companies?
In today’s digital era, data obtained from various sources such as online transactions, social media, sensors, and other devices, provides a great opportunity for companies to improve their performance. Here are some of the main benefits of data analytics for companies:
1. Improve Decision Making
With data analytics , companies can make decisions that are more based on facts and data rather than guesswork or intuition alone. For example, in terms of marketing strategy, companies can analyze consumer behavior to tailor more targeted campaigns.
2. Improve Operational Efficiency
Data analytics can help companies identify areas that need improvement or cost reduction. For example, by analyzing a production process, a company can find steps that waste time or resources, and then make improvements.
3. Improve Customer Experience
Through data analytics , companies can identify trends and patterns in customer behavior, including product preferences and the best time to make a purchase. This allows companies to provide more personalized products and services, increasing customer satisfaction.
4. Develop a Better Business Strategy
In-depth data analysis allows companies to plan better business strategies. With accurate information about the market, competitors, and internal performance, companies can design more appropriate strategies to achieve long-term goals.
5. Reducing Risks and Identifying Opportunities
Through predictive analytics, companies can identify risks or potential problems before they occur. For example, in the financial sector, data analytics can be used to predict the possibility of bankruptcy or financial loss. In addition, data analytics also helps in identifying new market opportunities that were previously unseen.
Also Read: Switch Devices
Stages of Doing Data Analytics
The data analytics process generally consists of several stages that allow data to be processed and analyzed effectively. Here are the stages in conducting data analytics that are commonly used in many industries:
1. Data Collection
The first stage is to collect data that is relevant to the problem or analysis objective to be achieved. Data can come from various sources, such as:
- IoT ( Internet of Things ) sensors or devices
- Business or e-commerce transactions
- Social or public media
- Surveys or questionnaires to understand customer opinions
At this stage, it is important to ensure that the data collected is clean and relevant to the purpose of the analysis.
2. Data Cleaning
The collected data is often imperfect, for example there are missing values or duplicate data. Therefore, data cleaning is an important process in data analytics that involves filtering and correcting errors in the data to ensure that the analyzed data is accurate and valid.
3. Data Exploration
At this stage, data analysts begin to explore the cleaned data to look for patterns or relationships that may exist. This process often involves the use of statistical tools or data visualization to help gain a deeper understanding of the data.
4. Data Analysis
After exploring the data, the next step is to conduct further analysis to find deeper insights. This can involve using a variety of techniques, such as:
- Regression analysis to find the relationship between variables.
- Clustering to find groups of similar data.
- Predictive analytics to predict future trends.
5. Presentation of Results (Data Visualization)
Presenting results in a visual form, such as a graph or dashboard, is essential to help decision makers understand the analysis findings. These visualizations help communicate insights clearly and effectively.
6. Decision Making and Implementation
Ultimately, the findings from data analytics are used to make better decisions. For example, companies can change marketing strategies based on consumer behavior analysis or improve business processes based on efficiency findings.
Also Read: What is Reboot?
Types of Analytics Data
In general, data analytics can be divided into several types, each of which has a different focus depending on the purpose of the analysis. Here is an explanation of the main types of data analytics :
1. Descriptive Analytics
Descriptive analytics is a type of analytics that focuses on explaining what has happened by analyzing historical data. This technique provides an overview of existing data, such as a company performance report or sales summary.
Example:
- Monthly sales report showing sales and revenue figures.
- Analyze customer data to find out who shops most frequently.
2. Diagnostic Analytics (Diagnostic Analytics)
Diagnostic analytics helps answer the question “why” something happened. Using deeper analysis techniques, diagnostic analytics tries to identify the cause of an event or pattern in the data.
Example:
- Why did sales drop in a particular month? Were there external factors such as competition or internal issues that caused the decline?
- Why does the number of customers filing complaints increase during a certain period?
3. Predictive Analytics
Predictive analytics is a type of analytics that uses historical data and statistical algorithms to predict future events or trends. It is widely used to forecast consumer behavior, sales, or even machine failures in an industry.
Example:
- Product demand prediction based on past sales data.
- Customer churn prediction based on service usage patterns.
4. Prescriptive Analytics
Prescriptive analytics provides recommendations for actions to be taken based on findings from descriptive and predictive analytics . It helps organizations make better decisions by providing insight into the options available and the potential impact of different actions.
Example:
- Recommendations on how to optimize your supply chain or production flow.
- Recommendations for personalizing marketing campaigns.
5. Causal Analytics
Causal analytics focuses on finding cause-and-effect relationships in data. This technique is used to understand the impact of certain actions or changes on outcomes.
Example:
- Analyze whether increased advertising spending leads to increased sales.
- Identifying factors that influence patient health after medical intervention.
Conclusion
Data analytics is a very powerful tool in the modern business and technology world. By leveraging data analytics , companies can gain deeper insights from the Big Data within their company, improve decision-making, identify new opportunities, and reduce risks. Whether it’s diagnostic analytics
Book References
- Davenport, T.H., & Harris, J.G. (2017). Competing on Analytics: The New Science of Winning (2nd ed.). Harvard Business Review Press.
This book discusses the importance of analytics in the modern business world and how companies can leverage data to gain a competitive advantage. - Provost, F., & Fawcett, T. (2013). Data Science for Business: What You Need to Know about Data Mining and Data-Analytic Thinking . O’Reilly Media. This book provides insight into how data analytics
concepts are applied in the business world, including techniques and strategies for improving decision making through data. - Shmueli, G., Patel, NR, & Bruce, PC (2016). Data Mining for Business Analytics: Concepts, Techniques, and Applications in R (2nd ed.). Wiley.
This book is excellent for understanding data analytics with a focus on the application of data mining techniques in business analysis using R. - Keller, S., & Tushman, M. L. (2016). The Innovator’s Dilemma: When New Technologies Cause Great Firms to Fail (Updated edition). Harvard Business Review Press.
While the focus is on technological innovation, the book also covers the role of analytics in helping companies adapt to technological and market changes. - Marr, B. (2016). Big Data in Practice . Wiley.
This book discusses how big data analytics is applied across industries and how organizations can use data to improve their operations.
Author: Elina Pebriyanti | Editor: Meilina Eka | Directorate of Information Technology Center